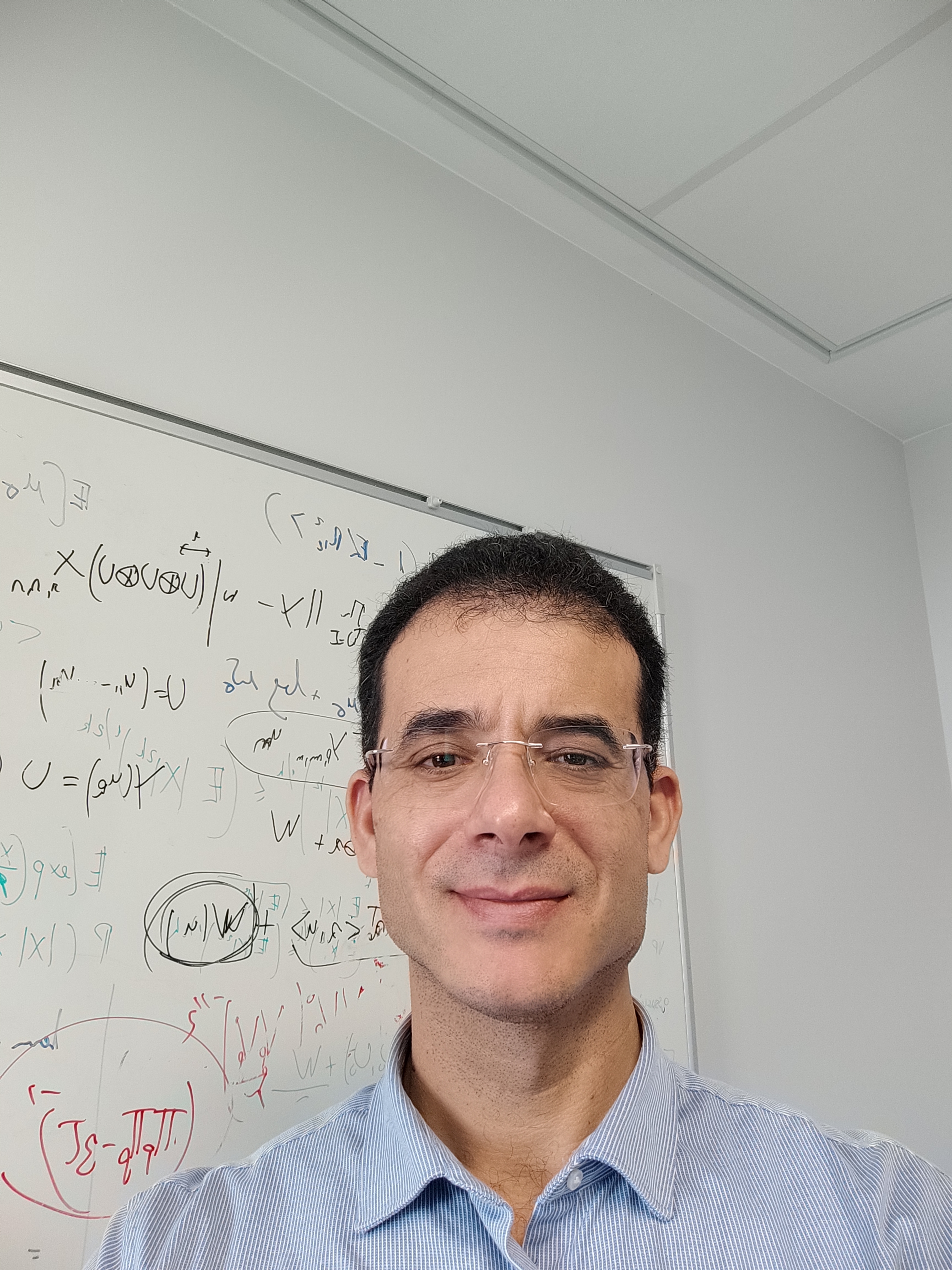
Abdellatif Zaidi
Associate Professor
Université Gustave-Eiffel
Navigation
Links
|
|
Homepage
News
- Paper "Heterogeneity Matters Even More in Distributed Learning Systems," by M. Kavian, M. Sefidgaran, A. Zaidi and R. Chor, submitted for publication at the IEEE International Symposium on Information Theory (ISIT 2025).
- Paper "Multi-View Representation Learning Regularizer With Gaussian-Product Mixture Prior," by M. Sefidgaran and A. Zaidi, submitted for publication at the IEEE International Symposium on Information Theory (ISIT 2025).
- Paper "Generalization Guarantees for Representation Learning via Data-Dependent Gaussian Mixture Priors ," by M. Sefidgaran, A. Zaidi and P. Krasnowski, selected to be presented as a SPLOTLIGHT at the Thirteenth International Conference on Learning Representations (ICLR 2025).
- Paper "Generalization Error of Federated Learning & Clients-Server Communication: Bounds, Algorithms for their Computation and Implications," by M. Sefidgaran, R. Chor and A. Zaidi submitted to the Journal of Machine Learning Research (JMLR), Feb. 2025.
- Paper "Data-dependent generalization bounds via variable size compressibility," by M. Sefidgaran and A. Zaidi accepted for publication at the IEEE Transactions on Information Theory, Sep. 2024.
- Paper "Implicit Compressibility of Overparametrized Neural Networks Trained with Heavy-Tailed SGD," by Y. Wan and M. Barsbey and A. Zaidi and U. Simsekli, accepted for publication at the Fourty-first International Conference on Machine Learning (ICML 2024), Vienna, Austria
- Paper "Federated Learning: You may communicate less often !," by M. Sefidgaran, R. Chor, A. Zaidi and Y. Wan, accepted for publication at the Fourty-first International Conference on Machine Learning (ICML 2024), Vienna, Austria
Education
- Ph.D., TÉLÉCOM ParisTech, 2006
- M.S., TÉLÉCOM ParisTech, 2003
- B.S., ENSTA ParisTech, 2002
Research Interests
- Information theory
- Statistical learning theory
- Distributed learning systems
- Machine learning and applications
Teaching
|